Valley Bank Financial Crime Compliance with AI
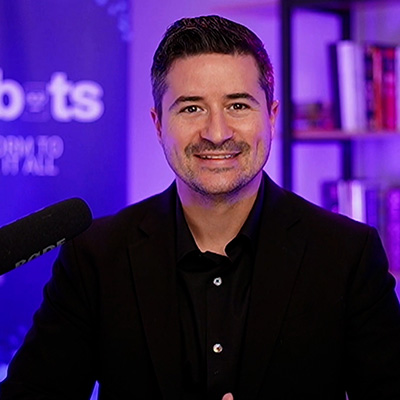
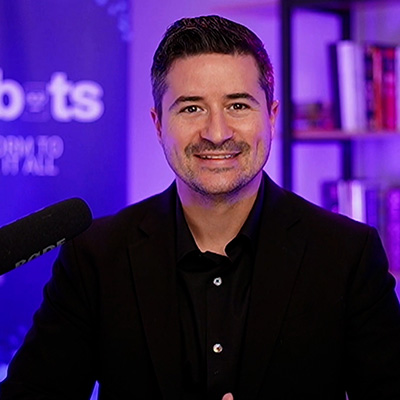
About Jason Dzamba
Director of Media Relations, Productivity Strategist, and Host of Inside the Bot Podcast, Jason uses a process-driven approach to help leaders optimize their actions and achieve their most important business objectives. His creative outlet is painting abstract art and producing music. He lives in Orlando, Florida, with his three kids.
Recent Posts
Related Blog Posts
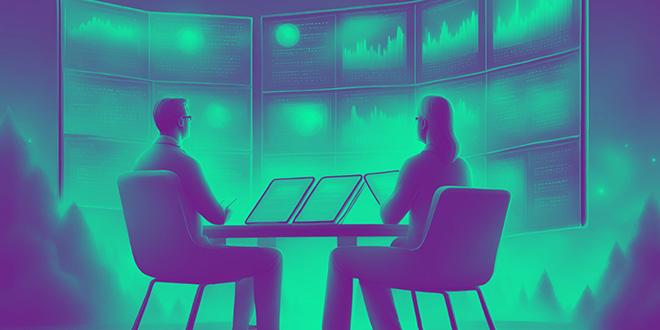
Innovation in Insurance: The Strategic Power of Data
The Deloitte AI Institute revealed a pivotal shift in the mindset of innovative insurance firms. Unlike their counterparts, these firms don’t treat data as a costly necessity or a byproduct of operations…
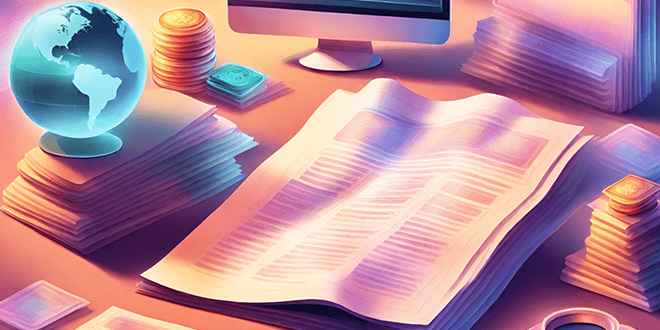
Automating Asset and Wealth Management Term Sheets with Enterprise AI
In this article, we’ll cover the practical outcomes of embracing AI, particularly in automating term sheets within platforms such as Salesforce…
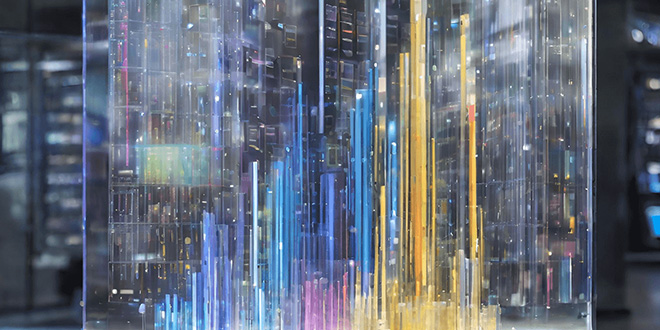
Fax Data Efficiency: Practical Healthcare Transformation
To give you practical aspects of healthcare transformation, we brought in Reno, a healthcare transformation data expert. His focus? The intake side and referral processes…